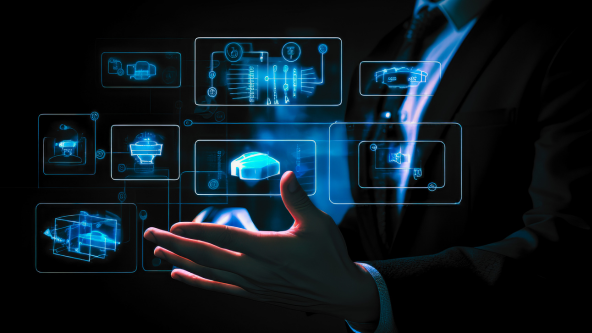
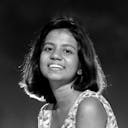
Transforming asset management with IBM Process Mining and Ennuviz
The 21st century has witnessed a paradigm shift to automated processes in close liaison with artificial intelligence or AI. While still widely regarded as ahead of time, AI is contemporaneous.
But is this change becoming a revolution? Is AI taking over? Sapiens have a lengthy history of being resentful of and hungry for change. But changes had us surviving through civilizations. In this blog, we'll look through the history of AI and its contemporary adeptness.
Before diving deep into the ongoing AI revolution, let's try to understand AI's origins and initial applications to comprehend better the quick shifts happening across industries.
As we recently recognized, AI doesn't just allude to AI software and tools. AI spawns beyond computers to robots and machines that date long back in the history of computers. The earliest known mentions of AI are in the
In 1952, the theory in papers was brought to practice through an AI program by Christopher Strachey to play the game of checkers.
So, how did that abrupt advancement occur from playing a game of checkers to writing codes?
Earlier, computers performed tasks automatically, and machines in industry were a subject of fiction. Humanoids, aliens, and even cars and airplanes were predicted in books centuries ago. For nearly sixty years, the AI concept has been in theory and was realized with the advent of computers.
The journey was extensive yet rewarding.
When the program was a success, research on AI continued, focusing on writing programs to solve logical and reasoning questions. The Logical Theorist was developed due to this quest by Allen Newell, Herbert A. Simon, and Cliff Shaw. It was the first program designed by humans to solve reasoning questions and theorems. The Logical Theorist proved theorems from Principia Mathematica, which was baffling in 1956.
As time went by, more research was conducted in the AI arena. In 1972, the first chatbot, similar to ChatGPT, Eliza and Parry, came into existence. They were both programmed to play the roles of therapist and paranoid human. They could produce a vague human-like conversation with one another within their programmed persona.
This is also the first recorded AI dialogue where the seed for interactive AI was sown. These were the early days when humans saw the Narrow AI, which was limited and highly domain-specific in performing tasks.
Later in the century, machine learning, a part of AI, made headway to automation. The goal of AI has always been to create a replica of a human brain/human and to hand over tasks to it. In a way, with the recent advancements, AI has reached its envisioned place.
AI in the present has countless real-time applications every day. We've broken down some of them below to describe the current AI trends.
Besides being a stimulated conversationalist, AI has become a part of our everyday life. Unlike the previous tech revolutions, AI has taken over within a short time and was widespread among the masses, unlike the previous century technologies, like computers, which took more than a couple of decades to reach the commoner.
Hence, it can be inferred that AI is nothing similar compared to the previous revolutions humanity has encountered.
Assistants like Google Assistant, Alexa, and Siri are AI-powered. They now assist us with our diet and medications, play music for us, talk to us, answer our questions, and sometimes even manage our entire day. Assistants don't just stop with helping users; they control thermostats, adjust lighting, operate televisions and music systems, and integrate with mobile phones to maintain other home appliances like refrigerators and air conditioners.
They recognize the voice of users by using Natural Language Processing (NLP) and take instructions from users.
Computer Vision and face recognition are enabled in computers, tablets, laptops, and mobile phones by Convolutional Neural Networks (CNN), which recognize human faces and tell them apart.
Social media platforms like Facebook, Instagram, etc, and search engines like Google, Microsoft, Bing, etc, collect the digital activity and search history of each user using keywords, URLs, hashtags, interactions, location, and timeframe, analyze it, and predict the feed or search activity of users.
This is made possible using deep learning AI, a mesh of different neural networks, specifically Feed-forward Neural Networks (FNN), that predict what the user wants to read or view.
In the 1990s, the first e-commerce sites like Amazon and eBay came into business for manufacturers and small businesses to sell their products. These e-commerce websites have now developed into mobile applications. AI in e-commerce platforms for buyers personalizes the product recommendations for each user by studying their purchase history, product search habits, and input keywords. It also allows voice search and image recognition to fetch products for users.
Additionally, e-commerce sites offer 24/7 customer support to solve queries and answer questions, providing a fulfilling CX. AI in e-commerce uses a combination of both CNN and FNN.
If we talk about the possibilities of AI, they are endless, but if we talk about the potential of existing AI systems, they can go above and beyond.
Businesses have benefited greatly from each industrial and tech revolution of the millennia.
Almost all departments inside an operating business, like operations, finance, IT, risk management, and human resources, use AI to optimize business processes, digitize, and ensure high customer satisfaction. Some of the best use-case examples today are,
A holistic customer experience is what keeps a business alive and running. Streamlining customer experience is essential to keep users engaged and retain long-term customers.
Implementing AI chatbots (IVA-Intelligent Virtual Assistants) and voice assistance (IVR-Interactive Voice Response) to solve customer queries can personalize the customer experience and offer round-the-clock customer support throughout the year without interference.
Predicting customer behavior and following up after purchase increases the customer's satisfaction with the brand and retains them.
Data management is an extensive yet crucial task for businesses.
Data management involves gathering, filtering, integrating, and preserving information essential for businesses to function. Data in various formats from multiple sources are compiled and classified based on preferred criteria by firms. These gathered datasets can be used in decision-making.
AI and deep learning can ease the painstaking effort of researching and analyzing larger datasets. Deep learning AI and ML (Machine Learning) software devours large amounts of data and is later used for data extraction.
The most common use case of AI in database management is AI in medicine. Despite diagnosis and detection, AI has proven to be a successful transition from manual to digital record management. Clinical datasets must be precise and non-repetitive, as inaccurate records might stir up disarray. AI here helps data extraction for every patient, reads through their medical history, predicts the likelihood of any diseases, and warns patients or spots symptoms and patterns for diseases as a precaution.
Procurement is the backbone of the Supply Chain. Any fully functional business must have multiple suppliers and receive goods from different sources. Each supplier's risk level needs to be evaluated and addressed. Any agreement breach, payment failure, supplier no-shows, delays, and other operational disruptions are considered risks in supplier management. A strong procurement strategy is executed to maintain a smooth relationship with all business suppliers.
AI comes into play here, identifying the risk with each supplier and helping organizations choose the best supplier based on their organizational goals, budget, and needs.
Both large and small-scale industries can implement AI in supplier management. A use case of how AI can aid in supplier management is as follows.
To choose a supplier, the complete information of multiple suppliers, along with their pricing details and sales policies, are fed into the software. Based on the provided criteria by the buyer, AI segregates the data and chooses the best supplier. The criteria for selecting a supplier might differ with the size, goals, products, demands, and budget of the company, which is also fed into the system. Next, the concerned supplier data is consumed and appropriately analyzed to give a predictive analysis of the potential risks involved with each supplier. Once the supplier is finalized, drafting contracts, tracking supplier performance, and analyzing past data to choose suppliers in the future can be automated.
Manufacturing, transportation, and service industries invest heaps in machines. Energy sector, supply chain companies, logistics firms, mining companies, etc., rely on machines for their daily operations. With increasing demand and contention, the maximum productivity must be obtained from these units to accomplish business goals.
With deep learning technology, AI can study the complete prototype, working conditions, and history of a machine or equipment built along with its designated task. A myriad of data is scanned and ingested to predict possible faults and periodic maintenance appointments accurately. Going beyond cognitive and repetitive tasks currently being automated, a future Artificial Intelligence that can entirely replace human abilities might spring up, but that is a skeptical keynote.
AI does everything effortlessly, whether automating business processes, manufacturing, integrating processes, forecasting trends, optimizing costs, retail management, data mining, or creating workflows.
We currently live with an amalgamation of Narrow and General AI, with Narrow being deployed on a large scale.
But does that mean AI is ready to be left alone with all the work? This has been an intriguing question, and its answer can be vague. From a worker's focal point, it might seem that AI is being adopted swiftly. Still, in reality, the surge in AI adoption is creating a shortage of professionals to train, program, and deploy AI systems to perform activities.
Though AI algorithms are undeniably rampant and fast-paced, approaching the change we encounter with poise holds more reason than confronting the change, as this has always been the pursuit of AI research.
At present, we've embedded AI into almost all industries for better performance, eliminating rudimentary practices. Receding from conventional approaches has levitated workers' fear of being replaced and vulnerable. But currently, we've seen scientists advance to General AI, which equals the human mind in reasoning, logic, etc. We're yet to see superintelligence, which surpasses human intelligence and may or may not be an ethical threat to humanity, but we currently have staunch control over them. At this point, we've come too far to lean back; we've no choice but to take lessons from history. As a matter of fact, history has a habit of repeating itself, whether it's war or technology.
What the AI revolution has in reserve is unknown but can be replaced. To name the replacement, we must live through it, either swaying or assisting.
We at Ennuviz focus on transforming your business with RPA (Robotic Process Automation). In this digital age, we assist businesses in surviving and thriving in the dynamic digital sphere. We've been in the automation industry for over five decades, with an astounding profile of prominent firms as clients. We specialize in expert strategy consulting, advisory services, process mapping, automation, process management, and complete on-call support from our team. At Ennuviz, we offer complete automation of your business, offering analytics, operating, process mining, and services with complete insights into your firm's operations.
Transforming asset management with IBM Process Mining and Ennuviz
Data and Artificial Intelligence: Impact on the Healthcare Industry with UiPath
Usage Of Robotic Process Automation During Covid 19
Because we put you first. Our customer-obsessed working model honors client's needs.
Schedule Demo